Bioinformatics Data Analysis Pipeline- Spatial Transcriptomics
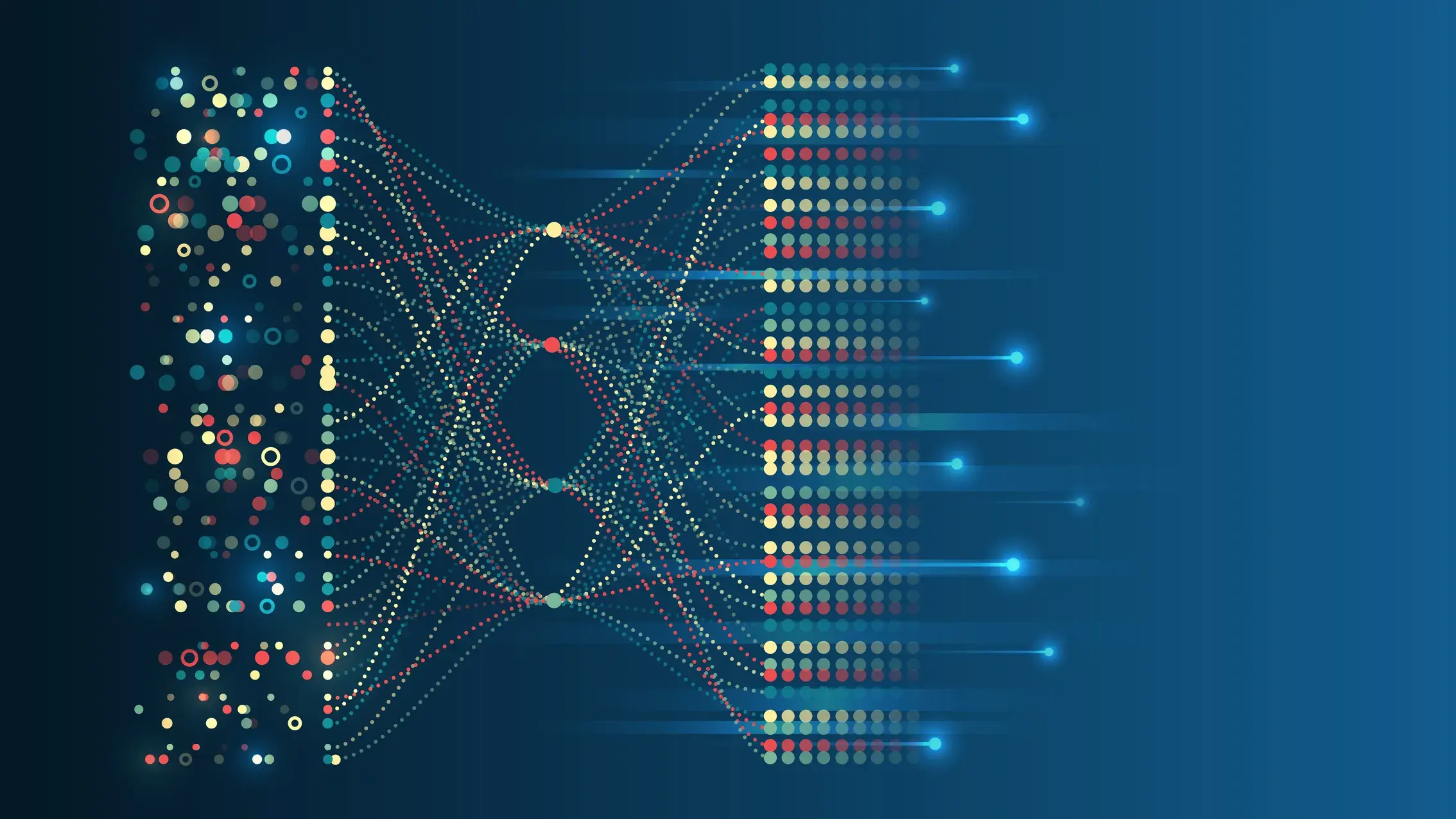
Bioinformatics Data Analysis Pipeline- Spatial Transcriptomics
-
Space Ranger
Space Ranger is a set of analysis pipelines that process 10x Genomics Visium data with brightfield or fluorescence microscope images, allowing users to map the whole transcriptome in a variety of tissues. Space Ranger v3.0 now supports Visium HD.
Reference: PMID: 28091601
Contact: Qin Ma
-
BayesSpace
A fully Bayesian statistical method that uses the information from spatial neighborhoods for resolution enhancement of spatial transcriptomic data and for clustering analysis.
Reference: PMID: 34083791
Contact: Qin Ma
-
SPRUCE
A Bayesian spatial multivariate finite mixture model based on multivariate skew-normal distributions, which is capable of identifying distinct cellular sub-populations in HST data.
Reference: PMID: 35895854
Contact: Qin Ma
-
SpaGCN
A graph convolutional network approach that integrates gene expression, spatial location and histology in SRT data analysis. Through graph convolution, SpaGCN aggregates gene expression of each spot from its neighboring spots, which enables the identification of spatial domains with coherent expression and histology.
Reference: PMID: 34711970
Contact: Qin Ma
-
SPARK-X
A non-parametric method for rapid and effective detection of spatially expressed genes in large spatial transcriptomic studies.
Reference: PMID: 34154649
Contact: Qin Ma
-
RCTD
A computational method that leverages cell type profiles learned from single-cell RNA-seq to decompose cell type mixtures in spatial transcriptomic while correcting for differences across sequencing technologies.
Reference: PMID: 33603203
Contact: Qin Ma
-
CARD
A deconvolution method that combines cell-type-specific expression information from single-cell RNA sequencing (scRNA-seq) with correlation in cell-type composition across tissue locations.
Reference: PMID: 35501392
Contact: Qin Ma
-
SpaTalk
A graph network and knowledge graph to model and score the ligand-receptor-target signaling network between spatially proximal cells by dissecting cell-type composition through a non-negative linear model and spatial mapping between single-cell transcriptomic and spatially resolved transcriptomic data.
Reference: PMID: 35908020
Contact: Qin Ma
-
COMMOT
It can infer cell–cell communication in spatial transcriptomics, which accounts for the competition between different ligand and receptor species as well as spatial distances between cells.
Reference: PMID: 36690742
Contact: Qin Ma