Image Data Analytics
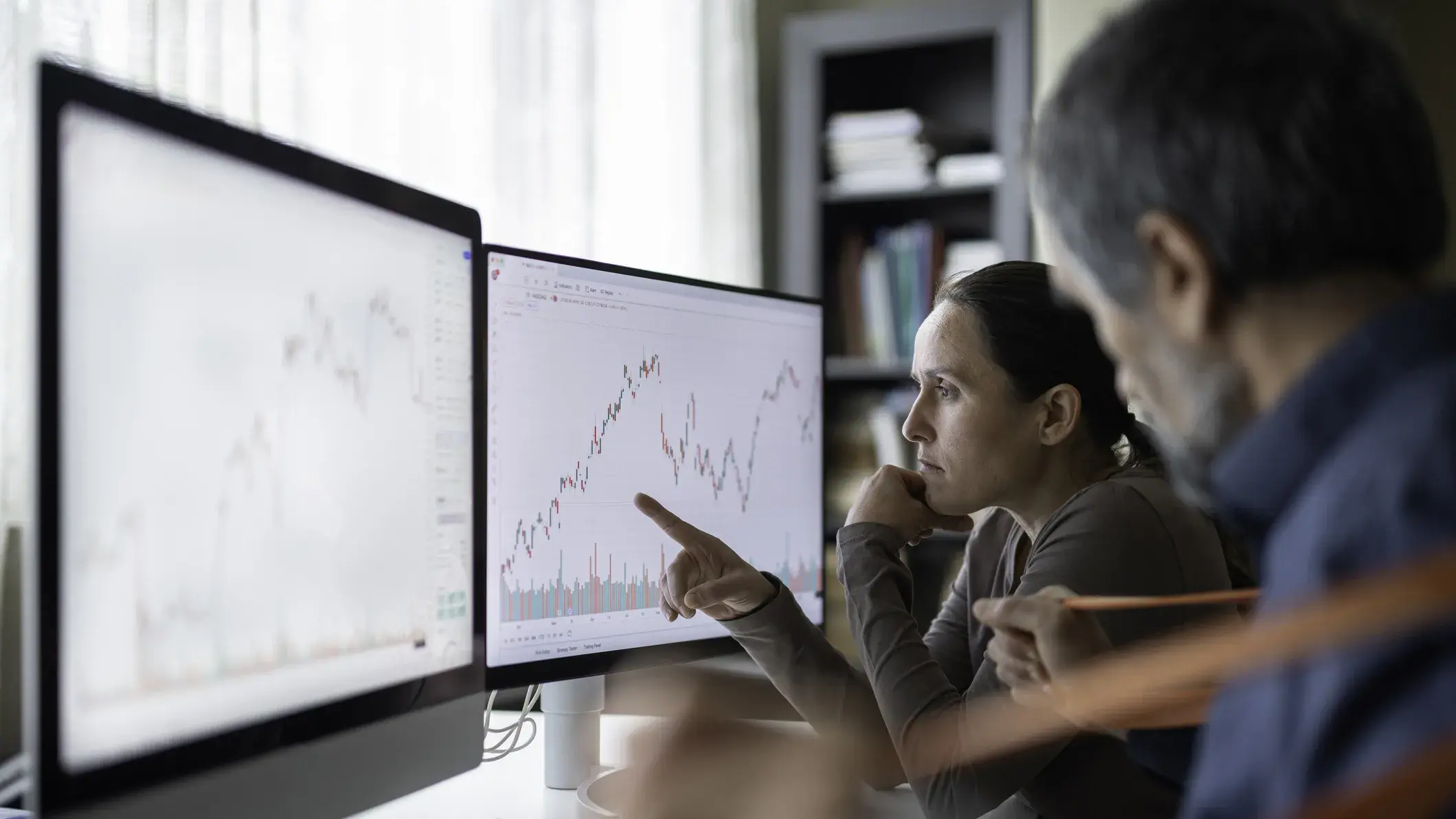
Image Data Analytics
-
ITK-SNAP
ITK-SNAP is an open-source software application for medical image segmentation. It features manual, semi-automatic and automatic tools for delineating anatomical structures in 3D imaging data.
Reference: https://doi.org/10.1016/j.neuroimage.2006.01.015
Contact: Yuan Xue
-
ANTs
ANTs is a suite of image processing tools for medical image analysis, including image registration, segmentation and statistical analysis. It is widely used for brain imaging studies and other biomedical applications.
Reference: https://doi.org/10.1038/s41598-021-87564-6
Contact: Yuan Xue
-
3D Slicer
3D Slicer is a free, open-source software platform for the analysis and visualization of medical images. It supports a variety of image processing tasks, including segmentation, registration and 3D reconstruction.
Reference: PMID: 22770690
Contact: Yuan Xue
-
Segment Anything Model 2 (SAM 2)
SAM 2 is a general-purpose, promptable segmentation model that can be used to segment objects in images and videos. Developed by Meta AI, it is designed to handle a wide variety of image types and segmentation tasks with minimal manual annotation.
Reference: ArXiv: 2408.00714
Contact: Yuan Xue
-
QuPath
QuPath is an open-source software for digital pathology image analysis. It provides tools for the visualization, annotation, and analysis of whole slide images, with a focus on machine learning-based methods for tissue and cell classification.
Reference: https://doi.org/10.1038/s41598-017-17204-5
Contact: Yuan Xue
-
ImageJ
ImageJ is a public domain, Java-based image processing program developed at the National Institutes of Health (NIH). It is widely used for scientific image analysis and supports a wide range of plugins for various types of image processing tasks.
Referenece: https://doi.org/10.1186/s12859-017-1934-z
Contact: Yuan Xue
-
MONAI (Medical Open Network for AI)
MONAI is an open-source framework designed for deep learning in healthcare imaging. It provides domain-optimized foundational capabilities for developing AI models, including pre-built pipelines for segmentation, classification and other image analysis tasks.
Reference: ArXiv: 2211.02701
Contact: Yuan Xue
-
nnU-Net
nnU-Net is a self-adapting framework for biomedical image segmentation. It automatically configures itself to a given dataset, optimizing the architecture, preprocessing and training pipelines. It has been highly successful in various biomedical image segmentation challenges.
Reference: https://doi.org/10.1038/s41592-020-01008-z
Contact: Yuan Xue